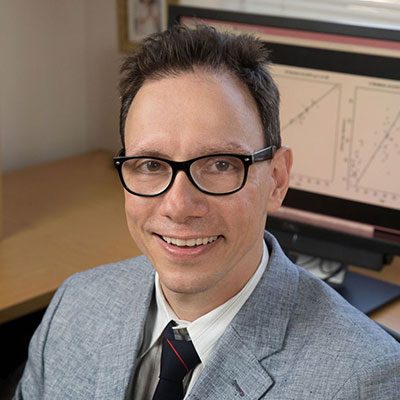
Stefan Horvath
Professor, Human Genetics, University of California Los Angeles
Professor, Psychiatry and Biobehavioral Sciences, University of California Los Angeles
Publications
- Horvath S, Haghani A, Zoller JA, Raj K, Sinha I, Robeck TR, Black P, Couzens A, Lau C, Manoyan M, Ruiz YA, Talbott A, Belov K, Hogg CJ, Sears KE. Epigenetic clock and methylation studies in marsupials: opossums, Tasmanian devils, kangaroos, and wallabies.. GeroScience, 2022.
- Horvath S, Lin DTS, Kobor MS, Zoller JA, Said JW, Morgello S, Singer E, Yong WH, Jamieson BD, Levine AJ. HIV, pathology and epigenetic age acceleration in different human tissues.. GeroScience, 2022.
- Horvath S, Haghani A, Peng S, Hales EN, Zoller JA, Raj K, Larison B, Robeck TR, Petersen JL, Bellone RR, Finno CJ. DNA methylation aging and transcriptomic studies in horses.. Nature communications, 2022.
- Horvath S, Haghani A, Zoller JA, Naderi A, Soltanmohammadi E, Farmaki E, Kaza V, Chatzistamou I, Kiaris H. Methylation studies in Peromyscus: aging, altitude adaptation, and monogamy.. GeroScience, 2021.
- Horvath S, Zoller JA, Haghani A, Lu AT, Raj K, Jasinska AJ, Mattison JA, Salmon AB. DNA methylation age analysis of rapamycin in common marmosets.. GeroScience, 2021.
- Horvath S, Raj K. DNA methylation-based biomarkers and the epigenetic clock theory of ageing.. Nature reviews. Genetics, 2018.
- Horvath S, Gurven M, Levine ME, Trumble BC, Kaplan H, Allayee H, Ritz BR, Chen B, Lu AT, Rickabaugh TM, Jamieson BD, Sun D, Li S, Chen W, Quintana-Murci L, Fagny M, Kobor MS, Tsao PS, Reiner AP, Edlefsen KL, Absher D, Assimes TL. An epigenetic clock analysis of race/ethnicity, sex, and coronary heart disease.. Genome biology, 2016.
- Horvath S. Erratum to: DNA methylation age of human tissues and cell types.. Genome biology, 2015.
- Horvath S, Erhart W, Brosch M, Ammerpohl O, von Schönfels W, Ahrens M, Heits N, Bell JT, Tsai PC, Spector TD, Deloukas P, Siebert R, Sipos B, Becker T, Röcken C, Schafmayer C, Hampe J. Obesity accelerates epigenetic aging of human liver.. Proceedings of the National Academy of Sciences of the United States of America, 2014.
- Horvath S, Zhang B, Carlson M, Lu KV, Zhu S, Felciano RM, Laurance MF, Zhao W, Qi S, Chen Z, Lee Y, Scheck AC, Liau LM, Wu H, Geschwind DH, Febbo PG, Kornblum HI, Cloughesy TF, Nelson SF, Mischel PS. Analysis of oncogenic signaling networks in glioblastoma identifies ASPM as a molecular target.. Proceedings of the National Academy of Sciences of the United States of America, 2006.
- Horvath S, Xu X, Lake SL, Silverman EK, Weiss ST, Laird NM. Family-based tests for associating haplotypes with general phenotype data: application to asthma genetics.. Genetic epidemiology, 2004.
- Horvath S, Xu X, Laird NM. The family based association test method: strategies for studying general genotype--phenotype associations.. European journal of human genetics : EJHG, 2001.